
The researchers’ first recourse was to ask Schaefer to evaluate the predicted trajectories for hypothetical plays. Absent the ground truth about the plays’ outcome, how do you gauge the model’s performance? It was straightforward to calculate the model’s accuracy on plays that had actually taken place on NFL football fields: the researchers simply fed the model a sequence of three player position measurements and determined how well it predicted the next ten.īut one of the purposes of defender ghosting is to predict the outcomes of plays that didn’t happen, in order to assess players’ decision making. But the researchers’ job wasn’t done yet. That model proved quite accurate at predicting defensive backs’ trajectories. The AWS-NFL team chose the features most important to the gradient boosting model, and just those features, as inputs to the deep-learning model.
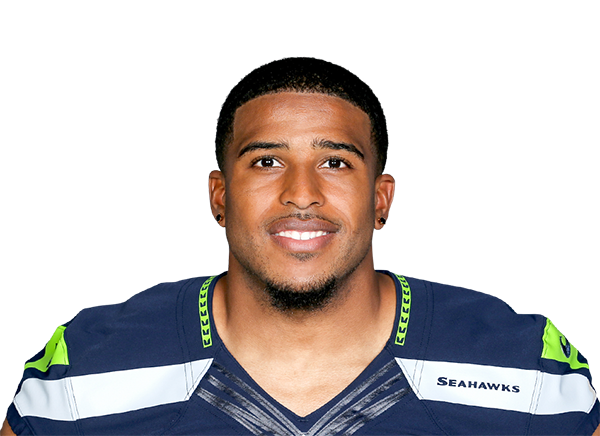
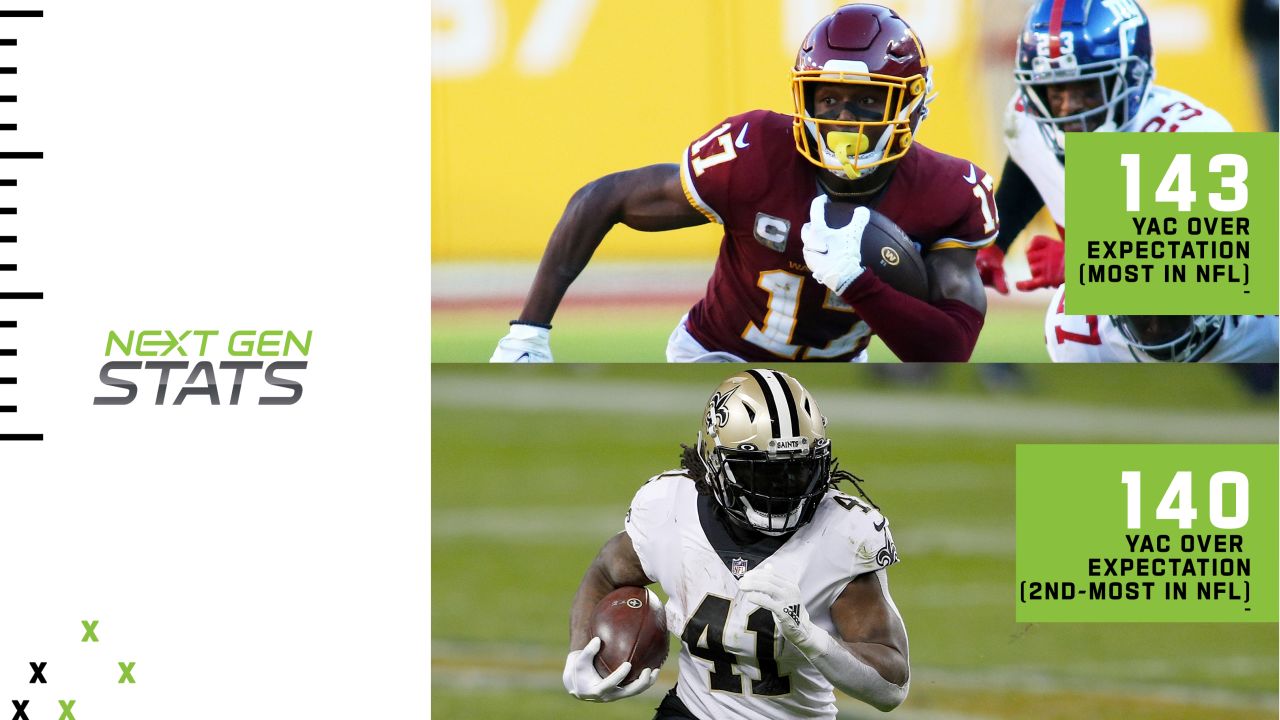
Gradient boosting models tend to be less accurate than neural networks, but they make it easy to see which input features make the largest contributions to the model output. But first they trained a simpler model, called a gradient boosting model, on all the available features. To predict trajectories, the AWS researchers planned to use a deep-learning model. Absent a huge amount of training data, it’s usually preferable to keep the feature set small. The more input features a machine learning model has, however, the more difficult it is to tease out each feature’s correlation with the phenomenon the model is trying to predict. But any number of other features - down and distance, distance to the goal line, elapsed game time, length of the current drive, temperature - could, in principle, affect player performance. For every player on the field, the NFL tracking system provides location, direction of movement, and speed, which are all essential for predicting defensive backs’ trajectories. Features are the different types of input data on which a machine learning model bases its predictions. Next, the team winnowed down the “feature set” for the model. Michael Schaefer, director of product and analytics for the NFL’s Next Gen Stats For instance, defender ghosting can help estimate how a play would have evolved if the quarterback had targeted a different receiver: would the defensive backs have reached the receiver in time to stop a big gain? Defender ghosting can thus help evaluate a quarterback’s decision making. Take, for instance, the problem of defender ghosting, or predicting the trajectories of defensive backs after the ball leaves the quarterback’s hand.ĭefender ghosting is not itself a Next Gen Stat, but it’s an essential component of stats under development. “And where we’ve needed additional ML expertise,” Schaefer adds, “AWS’s data scientists have been an invaluable resource.” Secondary variance
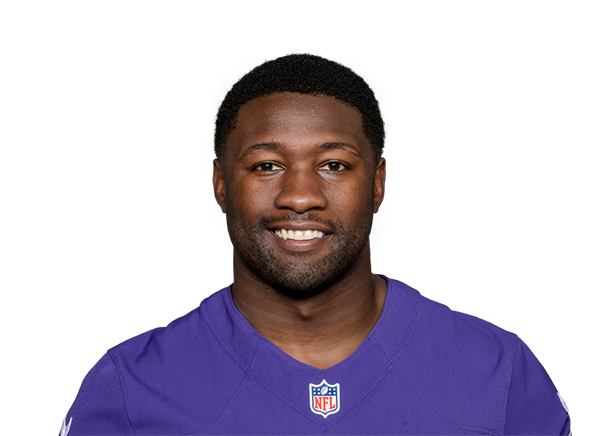
“SageMaker makes the development of ML models easy and intuitive - particularly for those who may not have deep familiarity with ML.” “We wouldn’t have been able to make the strides we have as quickly as we have without AWS,” says Michael Schaefer, the director of product and analytics for the NFL’s Next Gen Stats.
NFL STATS LEADERS SOFTWARE
AWS stores the huge amount of data generated by tracking every player on every play in every NFL game - nearly 300 million data points per season NFL software engineers use Amazon SageMaker to quickly build, train, and deploy the machine learning (ML) models behind their most sophisticated stats and the NFL uses the business intelligence tool Amazon QuickSight to analyze and visualize the resulting statistical data. Since 2017, Amazon Web Services (AWS) has been the NFL’s official technology provider in every phase of the development and deployment of Next Gen Stats. Lin Lee Cheong and her colleagues presented their paper on defender ghosting at the 2021 MIT Sloan School Sports Analytics Conference.
